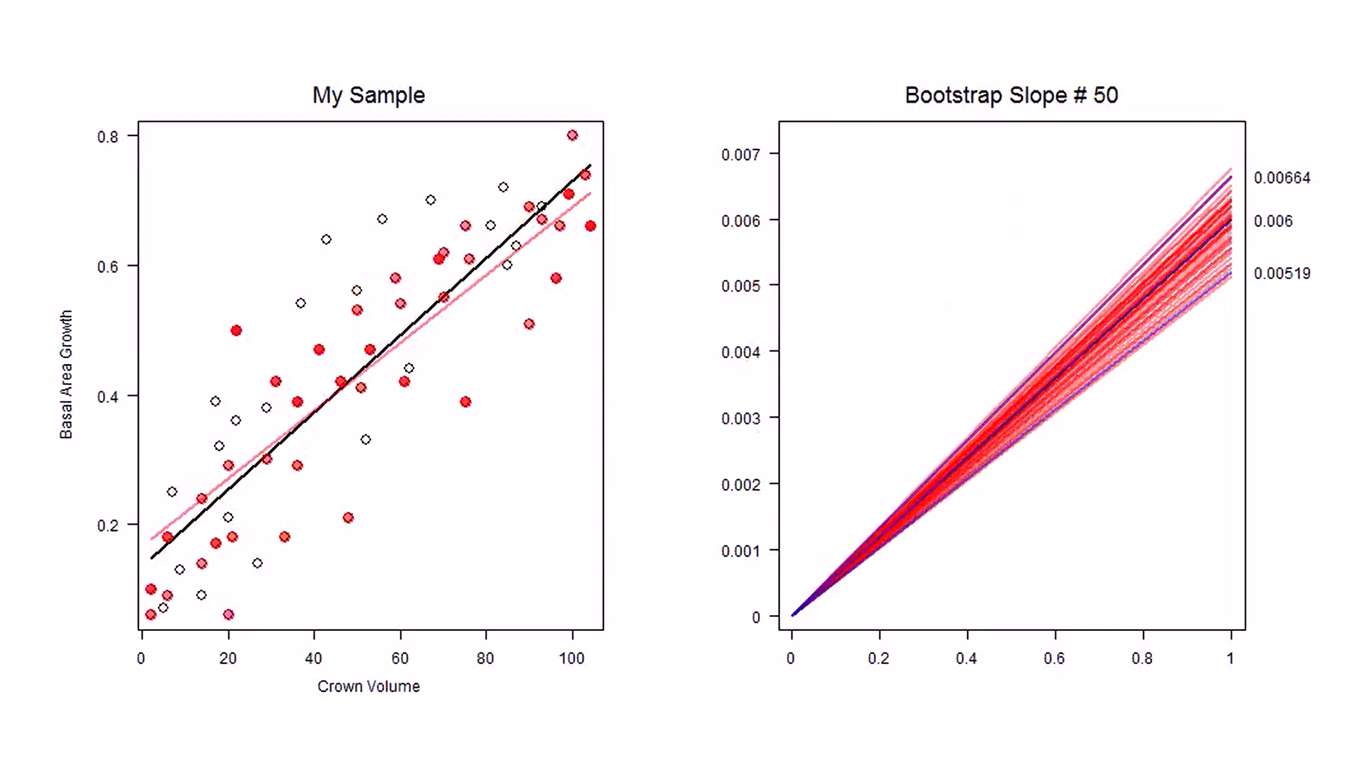
How we build robust MMM models with help of Bootstrapping
In statistics, especially inferential statistics, the corner stone paradigm is that of sample-population. We most often don’t have the population details. We hence try to infer things about the population through the sample. For e.g. Population parameter is estimated through sample statistic. ▪ What is Bootstrapping? Bootstrapping simply put is a method of repeated sampling (with replacement) of a sample. The sample chosen is assumed to be a good representation of the population. ▪ What is the utility of Bootstrapping? Bootstrapping should be seen as a method to learn about the sampling distribution rather than a method to estimate the population parameter. If we take simple linear regression as example,