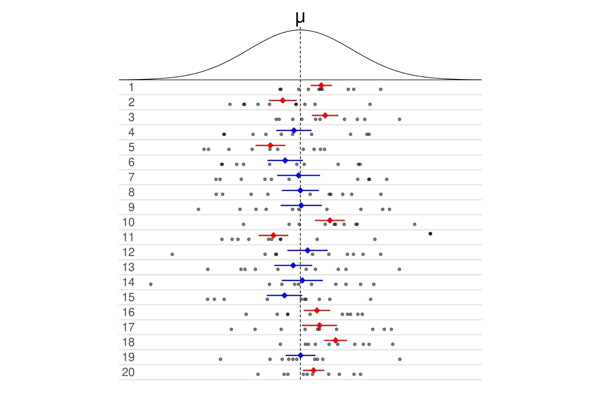
Confidence intervals in a way are barometer of your Marketing Mix Models
A lot of people blame Confidence Intervals and its ‘unintuitive’ nature for switching to Bayesian side of things. But if you are in Marketing Mix modeling domain, frequentist concept of confidence interval makes more sense. Before I elaborate, let me provide a quick recap of what exactly is Confidence Interval. 📌 What is confidence Interval? Lets say we are talking about the popular 95% CI. The definition of it would be – If one ran the same statistical test taking different samples and constructed a confidence interval each time, then in 95% of the cases, the confidence interval so constructed for that sample will contain the true parameter. Now that