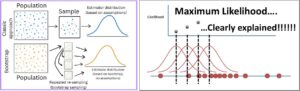
The MMM model speaks but the problem is we often don’t listen. Much like a poorly tuned guitar produces noise rather than good notes, your model too shows tell tale signs of poor fit.
So who are the truth whisperers?
I consider Maximum Likelihood Estimation and Bootstrapping as truth whisperers.
📌 Maximum Likelihood Estimation
Simply put maximum likelihood estimation tells you – Given your data, what values of the parameter are more likely to have generated the data.
It is simple but very elegant idea.
Why is it a truth whisperer?
In MMM, our goal is inference and to find the accurate attribution coefficients. MLE as a technique helps you narrow down on the parameter values that is more likely to have generated the data.
In MMM parlance you can think of it as – what values of your media / marketing variables are more likely to have generated the sales data.
Unlike Bayesian priors, you don’t start off with a bias. In MLE, the data speaks without any bias.
📌 Bootstrapping
Bootstrapping was invented by Bradley Efron. The idea was so simple that people initially thought it was ‘stupid’.
What is bootstrapping ?
Bootstrapping simply put is a method of repeated sampling (with replacement) of a sample. Of course the sample chosen is assumed to be a good representation of the population.
Why is it a truth whisperer?
Bootstrapping should be seen as a method to learn about the sampling distribution rather than a method to estimate the population parameter.
If we take simple linear regression as example, the model is fit to data and is used to make inferences about a larger population, hence the implicit assumption in interpreting regression coefficients is that the sample is representative of the population.
Generally, we don’t have access to the population quite often. All we have is the sample.
The question then arises about the quality of the sample and its estimate. How can we be sure of the coefficients in linear regression? Bootstrapping can provide information about the variability of the coefficients.
Bootstrapping can hence be used to construct confidence intervals.
In MMM parlance, Bootstrapping gives you clues whether you have honed in on the true attribution coefficient of the marketing variable or true ROI of the marketing variable.
If your bootstrapped confidence intervals gives you widely varying ranges each time, then it is sign that your model is not a good fit.
In summary:
As a MMM analyst, make it a point to listen to MLE and Bootstrapping because they are the truth whisperers.
Image Credits : Bootstrapping (Justin Belair), Maximum Likelihood Estimation (Josh starmer). Full links in resources.
Resources:
Bootstrapping Image credits Justin Bélair – I would also urge readers to check out his post (https://www.linkedin.com/posts/justinbelair_biostatistics-causalinference-science-activity-7149143488004046849-y1ye?utm_source=share&utm_medium=member_desktop)
Maximum Likelihood Estimation Image credits (Joshua Starmer PhD) – I would highly encourage people to view his video on MLE (https://youtu.be/XepXtl9YKwc?si=COj-vDJvqYqGtQf4)
Also a link on how we use Bootstrapping in MMM :
https://www.arymalabs.com/how-we-build-robust-mmm-models-with-help-of-bootstrapping/
And a bit more detail on what is bootstrapping:
https://www.linkedin.com/posts/venkat-raman-analytics_statistics-datascience-machinelearning-activity-7064489468732346368-3OGt?utm_source=share&utm_medium=member_desktop