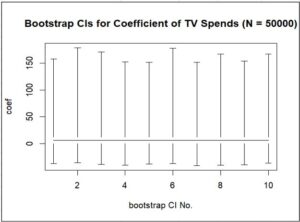
Experimentation has become a buzz word in MMM. Rightly so.
Experimentation like Difference in Difference (DID) can help one to holistically prove the efficacy of your MMM model. But, please save yourself the time and money and don’t do RCTs on MMM (check the link in resources to know why).
Coming to today’s topic, Experimentation is always considered post hoc (that is once the MMM model is built and finalized).
But what if we did experiments just after building a model? In a way to recalibrate the model.
Yes, there is a difference between calibration and validation of a model (check the link in resources).
So What Experiments can be done to calibrate models?
Ans: Bootstrapping.
In my last post I talked about how Bootstrapping as a truth whisperer in MMM. Because I didn’t want to pack in so much details, I left out some details.
You already learnt from my last post that Bootstrapping should be seen as a method to learn about the sampling distribution rather than a method to estimate the population parameter.
But one of the main purposes why Bootstrapping was invented was for Bias Correction.
I will first give a statistical explanation and then a Layman explanation.
π What exactly is a bias?
Bias is generally an attribute of the estimator.
Statistically speaking, Bias is the difference between estimator’s expected value and the true value of the parameter it is estimating.
An estimator is said to be unbiased if itβs expected value is equal to the parameter that weβre trying to estimate.
Now that the geeky explanation is over, lets unpack what this feature of unbiasedness means in MMM.
π Unbiasedness feature in MMM
MMM is all about attribution. There is a true value or true ROI of the marketing variable. Through MMM, our job is to hone in on this true ROI.
Our MMM models hence have to be unbiased so that we converge to this true ROI values.
How do we know if we have an unbiased model?
Ans: Bootstrapping again.
Bootstrapping gives you clues whether you have honed in on the true attribution coefficient of the marketing variable or true ROI of the marketing variable.
If your bootstrapped confidence intervals gives you widely varying ranges each time, then it is sign that your model could be biased.
It is important to get the model calibration right and bootstrapping can greatly help in this regard. This experimentation is cheap and effective too.
This bootstrapping experimentation can also inform you whether you should be doing validation experimentation like DID or not. Thus saving a lot of time and money.
π Pro tip: Use bias corrected bootstrapping for accurate results. The image is an example of bootstrapping confidence intervals for TV spends variables.
Uncertainty can be quantified through Frequentist methods too π .
π In summary: Carry out calibration experimentation first and then validation experimentation.
Resources:
DID paper:
https://www.arymalabs.com/proving-efficacy-of-mmm-through-difference-in-difference-did/
Calibration vs Validation :
https://www.arymalabs.com/calibration-vs-validation-in-mmm/
Don’t RCT MMM:
https://open.substack.com/pub/arymalabs/p/why-you-cant-rct-marketing-mix-models?r=2p7455&utm_campaign=post&utm_medium=web
Maximum Likelihood Estimation and Bootstrapping – The Truth Whisperers in Marketing Mix Modeling (MMM)
https://www.linkedin.com/posts/venkat-raman-analytics_marketingmixmodeling-statistics-marketingattribution-activity-7162692629187473409-ORvW?utm_source=share&utm_medium=member_desktop
Bias corrected CI:
https://blogs.sas.com/content/iml/2017/07/12/bootstrap-bca-interval.html