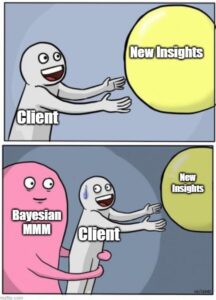
In MMM, everybody talks about incrementality. How to discern the incremental sales (or any KPI).
But what one should also question is – how much incremental insights one is getting out of MMM?
Has the MMM exercise informed you something extra that you did not know already?
We recently had a conversation with one client and here is a paraphrased excerpt of it
Client : “Why is that MMM sometimes gives us answers that we already know?”
Me: “Let me guess, your earlier vendor used Bayesian MMM ?”
Client : “yes, we were using Bayesian MMM and we actively helped the vendor in setting priors”
Me: “ah I know what the problem is”
The client later on lamented on how they have paid lots of money and practically got to know ‘what they knew already’.
They knew the marketing effectiveness of some channels but they didn’t get incremental information on other channels. Some of the channels didn’t even feature in the model !!
So what is the issue here?
๐๐ก๐ ๐ฉ๐ซ๐ข๐จ๐ซ๐ฌ
The biggest Achilles heel of Bayesian MMM are priors. Because of which one always starts off on the wrong foot (pun intended ๐ )
MMM is relatively a small data problem. This means that one never actually has enough data to overpower the biased priors one is setting.
The result – Your priors overpower the data, and in the output, you get what you knew already !!
So it shouldn’t be surprising that the model is stating the obvious.
I would highly urge readers to read Nuno Reis’ posts of Bayesian Thinking (Link in resources).
In his latest post he says “While Bayesian Thinking refines our beliefs with ‘tangible evidence’, it CONFINES us to ‘what is possible’ โ within what is already understood and quantified.”
In a MMM setting, the prior defining exercise is more or less about ‘what the marketing effectiveness of a marketing variable should be’. It in a way confines us to ‘what is possible’.
๐๐ฎ๐ญ ๐ฐ๐ก๐๐ญ ๐๐๐จ๐ฎ๐ญ ๐ ๐ซ๐๐ช๐ฎ๐๐ง๐ญ๐ข๐ฌ๐ญ ๐๐๐ , ๐๐จ๐๐ฌ ๐ข๐ญ ๐ง๐จ๐ญ ๐ก๐๐ฏ๐ ๐ญ๐ก๐ ๐ฉ๐ซ๐จ๐๐ฅ๐๐ฆ ๐จ๐ ๐ฌ๐ญ๐๐ญ๐ข๐ง๐ ๐ญ๐ก๐ ๐จ๐๐ฏ๐ข๐จ๐ฎ๐ฌ?
In short No. Frequentist MMM allows data to talk. There is no stifling of the voice of the data through priors. See my post on Truth whisperers in MMM (link in resources).
Of course any skilled statistician can also manipulate frequentist MMM to state the obvious or what client already knew. But the tools to do so are limited.
Bayesian MMM on the other hand provides a plethora of tools and opportunities to manipulate MMM. See my post on the same in resources.
Of late we are seeing a lot of Bayesian MMM adopters switch to us because of the above stated problem and more (See link in resources).
๐๐ง ๐ฌ๐ฎ๐ฆ๐ฆ๐๐ซ๐ฒ :
Don’t fall into the pitfall of knowing the obvious. Bayesian MMM will inevitably lead you to that. It is more prudent to adopt Frequentist MMM.
Resources:
Nuno Reisย post :ย https://www.linkedin.com/posts/nuno-reis_nunopost-activity-7170681948971986944-xtuR?utm_source=share&utm_medium=member_desktop
Bayesian MMM vs Frequentist MMM – Key Comparisons
https://www.linkedin.com/posts/venkat-raman-analytics_frequentist-mmm-vs-bayesian-mmm-comparison-activity-7161591828285210624-qyUm?utm_source=share&utm_medium=member_desktop
Which technique provides for great manipulation in MMM – Bayesian or Frequentist?
https://www.linkedin.com/posts/venkat-raman-analytics_marketingmixmodeling-statistics-marketingattribution-activity-7156533790130003968-qVTr?utm_source=share&utm_medium=member_desktop
Want performance guarantees ? choose Frequentist MMM.
https://www.linkedin.com/posts/venkat-raman-analytics_marketingmixmodeling-statistics-marketingattribution-activity-7151460386980945920-H-4U?utm_source=share&utm_medium=member_desktop
Adopting MMM for the first time ? Use Frequentist MMM.
https://www.linkedin.com/posts/venkat-raman-analytics_marketingmixmodeling-marketingattribution-activity-7148928932862472192-ozrW?utm_source=share&utm_medium=member_desktop